Research - Modern Phytomorphology ( 2021) Volume 15, Issue 4
Modeling stevia yields depending on plant density and mineral fertilizers rates
Raisa Vozhehova1, Pavlo Lykhovyd1*, Iryna Biliaieva1, Vitaliya Shebanova2, Oleksandr Rudik3 and Andrii Sinhaievskyi12Kherson State University, Universytetska Street 27, Kherson, 73003, Ukraine
3Kherson State Agrarian and Economic University, Stritenska Street 23, Kherson, 73006, Ukraine
Pavlo Lykhovyd, Institute of Irrigated Agriculture of NAAS, Naddniprianske, Kherson, 73483, Ukraine, Email: pavel.likhovid@gmail.com
Received: 07-Jul-2021 Accepted: 19-Jul-2021 Published: 26-Jul-2021, DOI: 10.5281/zenodo.5801191
Abstract
Stevia is one of the most prospective sweeteners and medicinal plants in dietic treatment. The crop is cultivated almost worldwide, however the cultivation technology is still studied insufficiently. The generalization of current achievements of Ukrainian and foreign scientists in the field of stevia cultivation was used as the basis for the creation of mathematical models of crop productivity depending on plant density and NPK fertilizers rates. The mathematical models, developed using the means of polynomial and multiple linear regression analysis, provided reasonable accuracy of the crop yield prediction (MAPE-28.77%-52.86%). The lowest errors (MAPE- 3.09%) were observed for the model of stevia productivity depending on plant density, which was created using an artificial neural network approach. It was determined that the best yields of stevia are obtained under the plant density of 80-120 thousand plant/ha and mineral nutrition with phosphorus and potassium fertilizers, while nitrogen ones are less important.
Introduction
The world market of sugary products reaches 80 billion USD, as estimated for 2011. 82 percent of the global market is occupied by sugar from sugar-beets and sugarcane, 9 percents are represented by sugary syrups, and the remaining 9 per cents falls on other sugar-replacing substances, among which stevia (Stevia rebaudiana Bertoni) is the prevailing source of sweeteners. While in the early 2000s, stevia’s main consumer was Japan (estimated as 90% of the total consumption), nowadays the products prepared using stevia raw material are in high demand worldwide, including such huge consumers as the USA, PRC, Germany, Russian Federation, Egypt, Indonesia, etc. Stevia’s popularity is also increasing in Ukraine (Roik et al. 2015).
Stevia is a well-known medicinal plant, which is used to produce natural sweeteners with high dietic value. Due to the content of a specific glycoside, built of glucose, sophorose, and steviol, the raw material of stevia is 300 times sweeter than conventional sugar. Besides, stevia contains many other health-giving substances, for example, phosphorus, calcium, copper, zinc, potassium, magnesium, chrome, selenium, vitamins A, C, E, some vitamins of the group B. Stevia is helpful in the management of glucose in the blood, arterial tension, obesity, etc. (Chernenko et al. 2013).
In Ukraine, the plant is cultivated in the farms for nearly 30 years, mainly in the Steppe and Forest-Steppe zones (Ermantraut & Stefaniuk 2019). However, until now, stevia remains an insufficiently studied medicinal plant, its cultivation technology is insufficiently developed and requires further studies (Ksenievych & Spryn 2018; Stefaniuk 2018). The main goal of our work was to accumulate, generalize and statistically process available data on the crop productivity in different conditions under a various number of plants per the area unit and fertilization peculiarities. Mathematical models of the crop productivity depending on these cultivation factors will help to find out the most prospective directions and variants to be included in the field experiments with the crop to distinguish the best options for the plant cultivation in the conditions of the South of Ukraine.
Materials and Methods
To create the models of stevia productivity depending on plant density and mineral fertilizers application rates we analysed the data of perennial studies, conducted during the recent decades in Ukraine and abroad (Aladakatti et al. 2012, Benhmimou et al. 2017, Shivani et al. 2019, Stefaniuk et al. 2015, Stefaniuk 2017, Stefaniuk & Bondarenko 2013, Taleie et al. 2012, Tansi et al. 2017, Ucar et al. 2018, Zavgorodniy 2003). The data of the studies was generalized and processed by the standard algorithm of multiple linear and polynomic regression analysis in Microsoft Excel 365 add-in BioStat v7 (Neter et al. 1996). Modelling stevia fresh biomass yield depending on plant population was also performed using an artificial neural network with 4 hidden neurons, which was trained for 1000 epochs at the learning rate of 0.80 by the back propagation algorithm. A neural network was implemented by Tiberius XL software (Brierley & Batty 1999). Evaluation of the created mathematical models was performed using the criterion of Mean Absolute Percentage Error (MAPE) and R2 values (Khair et al. 2017). The study is a part of the complex scientific investigation on the mathematical modelling of medicinal plant’s productivity (Lykhovyd et al. 2021).
Results
Polynomial regression analysis of the generalized experimental data of different studies allowed us to create the mathematical model of stevia productivity (in fresh biomass) depending on the plant’s population. Graphic analysis of the model points out that the maximum yield (on average, at the level of 22 t/ha-23 t/ ha) could be obtained in the crops with a density of 80-120 thousand plant/ha. The minimum productivity (10 t/ha- 15 t/ha) is usual for thinned crops (25-40 thousand plants/ ha), while excessive (over 120 thousand/ha) thickening of the plants leads to a slight drop in the yield (to 20-22 t/ ha). The highest yield of the vegetative mass of stevia at the level of 38.9 t/ha was obtained at a plant density of 100 thousand/ha, and the lowest one (7.0 t/ha) at a density of 40 thousand plants/ha (Fig. 1). The mathematical equation of the crop fresh biomass yield depending on the plant population is represented in the formula (1):
where Y-stevia fresh biomass yield, t/ha: x-plant density of the crop, thousands of plants/ha.
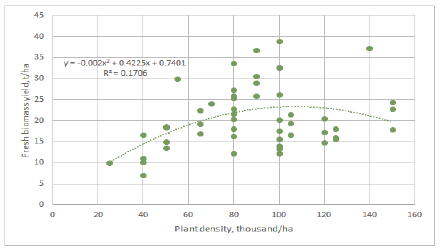
Figure 1: Approximation of the polynomial regression model for stevia fresh biomass yield depending on the plant density.
Statistics for model (1) are given in Tab. 1, testifying about the reasonable performance of the developed mathematical estimation method (Moreno et al. 2013).
Index | Value |
---|---|
R (correlation coefficient) | 0.4131 |
R2 (determination coefficient) | 0.1706 |
R2 (adjusted determination coefficient) | 0.1361 |
S (standard deviation) | 6.9775 |
MAPE (Mean Absolute Percentage Error) | 28.77 |
Zero hypothesis | Declined |
Table 1. Statistics for the model of stevia fresh biomass yield depending on plant density.
An artificial neural network model did not significantly outperform the regression one, although we must admit the lower errors of the model. The greatest disadvantage of the neural model is the absolute absence of the formula for its description and the impossibility of its use out of the Tiberius XL software environment. Some statistics for the model are presented in Tab. 2. Visualization of the neural network model for stevia productivity is presented in Fig. 2.
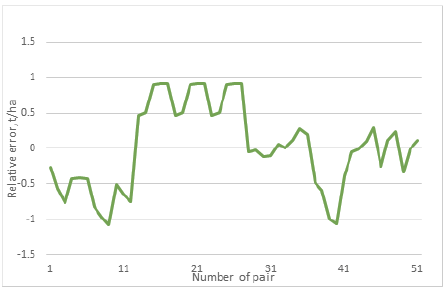
Figure 2: Relative errors of the model for stevia fresh biomass yield depending on plant density (artificial neural network computation).
Index | Value |
---|---|
R (correlation coefficient) | 0.3480 |
R2 (determination coefficient) | 0.1211 |
NE (normalized error) | 0.4857 |
MAPE (Mean Absolute Percentage Error) | 3.09 |
Table 2. Statistics for the model of stevia fresh biomass yield depending on plant density.
Besides, a mathematical model for stevia fresh biomass productivity depending on the rates of mineral NPK fertilizers was developed. It was found that mineral fertilizers have a positive effect on crop productivity. The model of the form (2) indicates that the most important nutrient for the crop is phosphorus (1 kg/ha of the applied active substance provides the formation of 28.7 kg/ha of fresh biomass of stevia), while the plant demands nitrogen are modest.
where Y-stevia fresh biomass yield, t/ha: NPK-mineral fertilizers application rates in the active substance, kg/ha.
Thus, stevia requires primarily phosphorus-potassium (and on soils with a high content of available potassiumonly phosphorus) nutrition to form high yields. Statistics of the developed mathematical model are given in Tab. 3. The model approximation has testified about a reasonable level of accuracy of the multiple regression equation (Moreno et al. 2013). The graphic model approximation is given in Fig. 3.
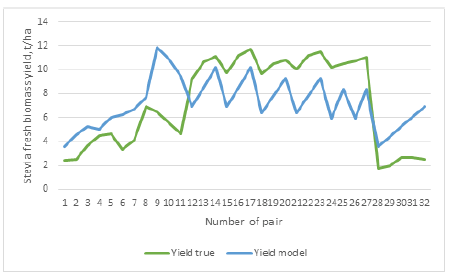
Figure 3: Approximation of the model of stevia fresh biomass yield depending on NPK fertilizers rates.
We are the first to develop and offer mathematical models of stevia productivity formation. Further studies will be targeted on the determination of the best options of sowing dates for the crop.
Conclusion
The results of the study revealed valuable regulations in stevia yield formation processes, namely: the best plant density for the crop is within the range of 80-120 thousand plant/ha, the necessity of mineral fertilization of the plant with a special focus on phosphorus fertilizers. Further studies are required to make theoretical knowledge about stevia yield formation deeper. Mathematical modelling is one of the most convenient, accurate and fast methods for better understanding the processes of productivity formation under the influence of different natural and anthropogenic factors.
References
Aladakatti Y.R., Palled Y.B., Chetti M.B., Halikatti S.I., Alagundagi S.C., Patil P.L., Janawade A.D. (2012). Effect of nitrogen, phosphorus and potassium levels on growth and yield of stevia (Stevia rebaudiana Bertoni). Karnataka J Agric Sci 25: 25-29. https://doi.org/10.2307/2399915
Benhmimou A., Ibriz M., Al Faïz C., Gaboun F., Douaik A., Amchra F.Z., Lage M. (2017). Effects of planting density and harvesting time on productivity of natural sweetener plant (Stevia rebaudiana Bertoni.) in Larache region, Morocco. Internat J Plant Res 7: 83-89. http://ofsq.everstevia.com/Dinsity-Harvesting-Time-Morocco.pdf
Brierley P., Batty B. (1999). Data mining with neural networks-an applied example in understanding electricity consumption patterns. Knowledge Discovery Data Mining 12: 240-303.
Chernenko O.V., Kormiltsev B.F., Kozlyk T.I. (2013). Peculiarities of stevia seed treatment before sowing and technology of seedlings cultivation in the conditions of Polissia. Agroinductrial Product Polissia 6: 74-77. http://dx.doi.org/10.11646/phytotaxa.257.2.6
Ermantraut E., Stefaniuk V. (2019). Biological and agrotechnical bases of stevia (Stevia rebaudiana Bertoni) cultivation in the Forest-steppe and the Steppe of Ukraine. Agrobiol 1: 47-54. https://doi.org/10.33245/2310-9270-2019-146-1-47-54
Khair U., Fahmi H., Al Hakim S., Rahim R. (2017). Forecasting error calculation with mean absolute deviation and mean absolute percentage error. In J Physics: Conf Series 930: 012002. https://iopscience.iop.org/article/10.1088/1742-6596/930/1/012002/meta
Ksenievych O.V., Spryn O.B. (2018). Cultivation of stevia in Kherson oblast. STEM-education as a direction for modernization in the learning methods of natural and mathematical disciplines at schools and higher educational establishments pp: 119-122.
Lykhovyd P.V. (2018). Prediction of sweet corn yield depending on cultivation technology parameters by using linear regression and artificial neural network methods. Biosyst Diversity 26: 11-15. https://doi.org/10.15421/011802
Lykhovyd P., Biliaieva I., Piliarskyi V., Lavrenko N., Maksymov M. (2021). Modeling valerian roots yield depending on mineral fertilization rates. M Phytomorphol 15: 51-55.
Moreno J.J.M., Pol A.P., Abad A.S., Blasco B.C. (2013). Using the R-MAPE index as a resistant measure of forecast accuracy. Psicothema 25: 500-506. https://doi.org/10.7334/psicothema2013.23
Neter J., Kutner M.H., Nachtsheim C.J. Wasserman W. (1996). Applied linear statistical models. 4th Edition WCB McGraw-Hill, New York.
Roik M., Kuznetchova I., Zaharevich V. (2015). Production and use of stevia (Stevia rebaudiana Bertoni) worldwide. Scientific Works of NUFT 25: 202-207.
Shivani K., Gautam G., Sukany G., MR M. (2019). Impact of spacing and levels of nitrogen on growth and yield of stevia (Stevia rebaudiana Bertoni). J Pharmacognosy Phytochem 8: 1878-1881. https://www.phytojournal.com/archives/2019/vol8issue3/PartX/8-2-461-151.pdf
Stefaniuk V.Yo. (2017). Effect of nutrition elements on the productivity of stevia. Sugar Beets 4: 18-19.
Stefaniuk V.Yo. (2018). Scientific basics of adaptive technology of growing stevia in Ukraine. Scientific Rep NULES Ukr 75.
Stefaniuk V.Yo., Bondarenko V.M. (2013). Productivity of stevia (Stevia rebaudiana Bertoni), depending on the plant population. Scientific Works Institute Bioenergetic Crops Sugar Beets 17: 300-302.
Stefaniuk V.Yo., Zhuzhalova T.P., Bondarenko V.M., Yendruzhiyevska L.P., Hura Yu.V. (2015). Determination of optimal plant density of the plants of stevia (Stevia rebaudiana Bertoni) under its seed propagation. Sugar Beets 3: 15-17.
Taleie N., Hamidoghli Y., Rabiei B., Hamidoghli S. (2012). Effects of plant density and transplanting date on herbage, stevioside, phenol and flavonoid yield of Stevia rebaudiana Bertoni. Intl J Agri Crop Sci 4: 298-302. https://www.researchgate.net/profile/Babak-Rabiei/publication/268006035_Effects_of_plant_density_and_transplanting_date_on_herbage_stevioside_phenol_and_flavonoid_yield_of_Stevia_rebaudiana_Bertoni/links/58e4ff8845851547e17e0af9/Effects-of-plant-density-and-transplanting-date-on-herbage-stevioside-phenol-and-flavonoid-yield-of-Stevia-rebaudiana-Bertoni.pdf
Tansi L.S., Samadpourrigani E., Gedik S. (2017). Effects of Different Plant Density and Cutting Times on Yield of Stevia under the Çukurova Conditions. Internatl J Secondary Metabolite 4: 355-358. https://doi.org/10.21448/ijsm.373822
Uçar E., Turgut K., ÖzyiÄ?it Y., Özek T., Özek G. (2018). The effect of different nitrogen levels on yield and quality of stevia (Stevia rebaudiana bert.). J Plant Nutrit 41: 1130-1137. https://doi.org/10.1080/01904167.2018.1431673
Zavgorodniy V. (2003). Cultural practices for increasing stevia yields under conditions of the Forest-Steppe zone of Ukraine. Collection of Scientific Works of the Institute of Sugar Beets of UAAS 5: 216-224.